Quantum-Inspired AI Compression Knowledge Exchange Workshop
Marketing
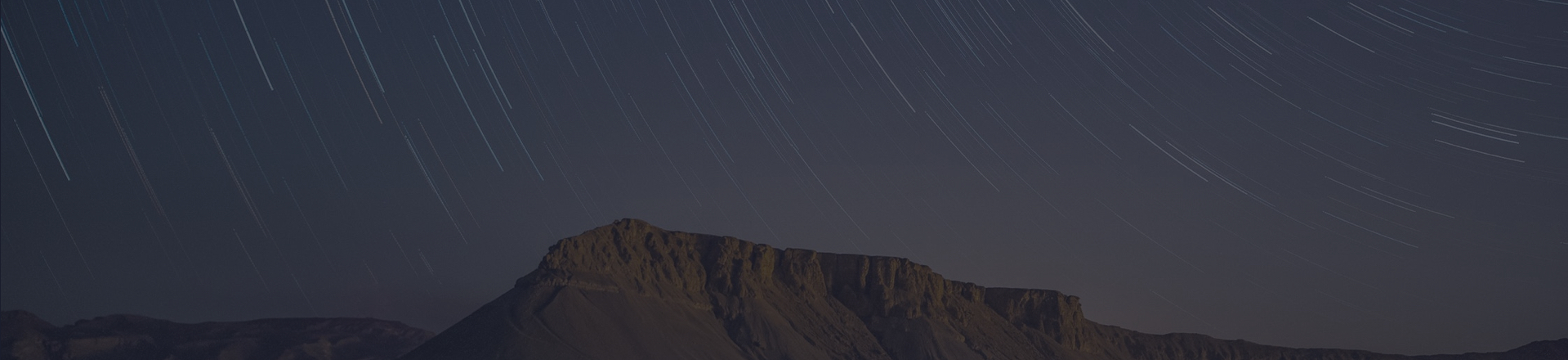
Following the successful Knowledge Exchange Sandpit at University College London (UCL) in October 2021, the Satellite Applications Catapult recently hosted a workshop with researchers from UCL and King’s College London (KCL), making use of UCL’s funding to support knowledge exchange between academia and industry.
The workshop was held at the Westcott Innovation Centre in Buckinghamshire in March 2022 and was focused on the compression of artificial intelligence (AI) models. Satellite companies worldwide are seeking to deploy AI models on-board their spacecraft which has the potential to offer faster access to analysis-ready data and to generate near-real-time insights rather than near-real-time data. However, the sizes and complexities of state-of-the-art AI models are continuously growing, making them unsuitable for running on computationally constrained satellites.
At the workshop were representatives from UCL, KCL, Deimos Space UK, D-Orbit, Little Place Labs, and the Satellite Applications Catapult. The workshop specifically sought to explore the use of novel compression techniques, developed by academic researchers at UCL and KCL and inspired by quantum physics, to shrink the size of state-of-the-art AI models. The workshop aimed to support the academic researchers in gaining a better understanding of the path towards on-board AI in satellite applications and the use-cases for on-board data processing, the technological developments needed to build these systems, and the technological roadblocks.
Mike Wilby (Deimos Space UK) said:
“Satellite on-board AI processing solutions are critical for enabling latency reductions in high priority Earth Observation services, such as extreme weather alerting and disaster response, and will enable insights to be delivered direct to locations where they are needed most. This promising new approach to deep learning model optimisation could remove a major barrier to delivering cutting-edge AI performance within resource-constrained environments.”
An overview of the application of the technology by the UCL and KCL researchers was provided. They covered an example problem they see their capability solving: pre-processing of raw image data is needed for analysis-ready data, on-board processing can reduce response times and lead to near-real-time insights, and link budgets can be stretched by large volumes of inadequate data which can be reduced through filtering by AI models. Drawing comparisons to the CloudScout project, using Sentinel-2 images to auto-label data and discard inadequate images, the researchers showed that their capability can reduce the size of the models by over 5 times, with no reduction in the accuracy of the model’s performance accuracy. They finally showed that off-the-shelf, state-of-the-art deep learning models with tens of thousands of hours of GPU hours of training (such as those found in Keras Applications, huggingface, tensorflow hub, pytorch hub, and timm, etc.) are currently too large to perform on satellites. But with their compression technique, they showed they could reduce the size by over 50% with no significant reduction in performance accuracy, enabling them to be used on-board satellites.
Some key insights gained during the exploration of use-cases and technology were that satellites are currently computationally constrained, but this will not always be the case as more sophisticated processors reach high technology readiness levels (TRLs). However, there will always be a need to perform computations faster and make models smaller, the opportunity will therefore remain to compress AI models. In addition, there is a small subset of use cases where satellite data is needed urgently, but these use cases are ideal candidates for on-board AI and therefore the model compression. There is also a constraint with uplinking new models and data to satellites, and in turn an opportunity for AI model compression to reduce the size of models being uplinked to satellites.
The main outcome of the workshop was that the external industry partners agreed to scope a collaborative project with the academic researchers, relating to the processing of data in a resource limited system. It was suggested that multiple AI models from Little Place Labs and Deimos Space (to analyse high-impact use-case data, e.g., on disaster responses, climate change, etc.) could be compressed using the quantum-inspired technique developed by the academic researchers and tested on-board a D-Orbit ION CubeSat carrier.
Following the workshop, Lewis Wright (KCL) and James Dborin (UCL) said:
“We have learnt the state of the current missions testing AI deployment in space, and what hardware and software options are available to satellite providers. Equipped with this knowledge, we firmly believe the novel compression techniques will be an asset to the space and satellite industry. Discussions are already underway with satellite AI model architects to deploy models in the very near future on platforms currently in orbit.”
Also, Mike Wilby (Deimos Space UK) said:
“Elecnor-Deimos is involved in emerging technology development and demonstration activities across multiple domains. We are interested to see how this technology may fit within this framework.”
This workshop demonstrates our ability to bring together different stakeholders to explore collaborative opportunities, bridging the gaps between academia and industry. For more information about our Knowledge Exchange work, visit our Working with Academia webpages.